Fast Rates for Regularized Least-squares Algorithm
Author(s)
Caponnetto, Andrea; Vito, Ernesto De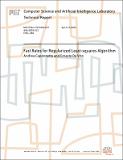
DownloadMIT-CSAIL-TR-2005-027.ps (15752Kb)
Additional downloads
Metadata
Show full item recordAbstract
We develop a theoretical analysis of generalization performances of regularized least-squares on reproducing kernel Hilbert spaces for supervised learning. We show that the concept of effective dimension of an integral operator plays a central role in the definition of a criterion for the choice of the regularization parameter as a function of the number of samples. In fact, a minimax analysis is performed which shows asymptotic optimality of the above-mentioned criterion.
Date issued
2005-04-14Other identifiers
MIT-CSAIL-TR-2005-027
AIM-2005-013
CBCL-248
Series/Report no.
Massachusetts Institute of Technology Computer Science and Artificial Intelligence Laboratory
Keywords
AI, optimal rates, regularized least-squares, reproducing kernel Hilbert space, effe