Some Properties of Empirical Risk Minimization over Donsker Classes
Author(s)
Caponnetto, Andrea; Rakhlin, Alexander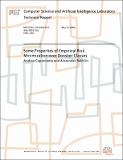
DownloadMIT-CSAIL-TR-2005-033.ps (6868.Kb)
Additional downloads
Metadata
Show full item recordAbstract
We study properties of algorithms which minimize (or almost minimize) empirical error over a Donsker class of functions. We show that the L2-diameter of the set of almost-minimizers is converging to zero in probability. Therefore, as the number of samples grows, it is becoming unlikely that adding a point (or a number of points) to the training set will result in a large jump (in L2 distance) to a new hypothesis. We also show that under some conditions the expected errors of the almost-minimizers are becoming close with a rate faster than n^{-1/2}.
Date issued
2005-05-17Other identifiers
MIT-CSAIL-TR-2005-033
AIM-2005-018
CBCL-250
Series/Report no.
Massachusetts Institute of Technology Computer Science and Artificial Intelligence Laboratory
Keywords
AI, empirical risk minimization, stability, empirical processes