Online Learning of Non-stationary Sequences
Author(s)
Monteleoni, Claire; Jaakkola, Tommi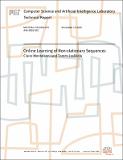
DownloadMIT-CSAIL-TR-2005-074.ps (9950.Kb)
Additional downloads
Metadata
Show full item recordAbstract
We consider an online learning scenario in which the learner can make predictions on the basis of a fixed set of experts. We derive upper and lower relative loss bounds for a class of universal learning algorithms involving a switching dynamics over the choice of the experts. On the basis of the performance bounds we provide the optimal a priori discretization of the switching-rate parameter that governs the switching dynamics. We demonstrate the algorithm in the context of wireless networks.
Date issued
2005-11-17Other identifiers
MIT-CSAIL-TR-2005-074
AIM-2005-032
Series/Report no.
Massachusetts Institute of Technology Computer Science and Artificial Intelligence Laboratory
Keywords
AI, online learning, regret bounds, non-stationarity, HMM, wireless networks