Rank Priors for Continuous Non-Linear Dimensionality Reduction
Author(s)
Stiefelhagen, Rainer; Darrell, Trevor; Urtasun, Raquel; Geiger, Andreas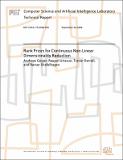
DownloadMIT-CSAIL-TR-2008-056.pdf (1.379Mb)
Additional downloads
Other Contributors
Vision
Advisor
Trevor Darrell
Metadata
Show full item recordAbstract
Non-linear dimensionality reduction methods are powerful techniques to deal with high-dimensional datasets. However, they often are susceptible to local minima and perform poorly when initialized far from the global optimum, even when the intrinsic dimensionality is known a priori. In this work we introduce a prior over the dimensionality of the latent space, and simultaneously optimize both the latent space and its intrinsic dimensionality. Ad-hoc initialization schemes are unnecessary with our approach; we initialize the latent space to the observation space and automatically infer the latent dimensionality using an optimization scheme that drops dimensions in a continuous fashion. We report results applying our prior to various tasks involving probabilistic non-linear dimensionality reduction, and show that our method can outperform graph-based dimensionality reduction techniques as well as previously suggested ad-hoc initialization strategies.
Date issued
2008-09-26Series/Report no.
MIT-CSAIL-TR-2008-056