Selecting Metrics to Evaluate Human Supervisory Control Applications
Author(s)
Cummings, M. L.; Pina, P. E.; Donmez, B.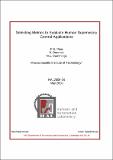
DownloadHAL2008-04.pdf (1.163Mb)
Other Contributors
Massachusetts Institute of Technology. Dept. of Aeronautics and Astronautics. Humans and Automation Laboratory
Metadata
Show full item recordAbstract
The goal of this research is to develop a methodology to select supervisory control metrics. This
methodology is based on cost-benefit analyses and generic metric classes. In the context of this research,
a metric class is defined as the set of metrics that quantify a certain aspect or component of a system.
Generic metric classes are developed because metrics are mission-specific, but metric classes are
generalizable across different missions. Cost-benefit analyses are utilized because each metric set has
advantages, limitations, and costs, thus the added value of different sets for a given context can be
calculated to select the set that maximizes value and minimizes costs. This report summarizes the
findings of the first part of this research effort that has focused on developing a supervisory control metric
taxonomy that defines generic metric classes and categorizes existing metrics. Future research will focus
on applying cost benefit analysis methodologies to metric selection.
Five main metric classes have been identified that apply to supervisory control teams composed
of humans and autonomous platforms: mission effectiveness, autonomous platform behavior efficiency,
human behavior efficiency, human behavior precursors, and collaborative metrics. Mission effectiveness
measures how well the mission goals are achieved. Autonomous platform and human behavior efficiency
measure the actions and decisions made by the humans and the automation that compose the team.
Human behavior precursors measure human initial state, including certain attitudes and cognitive
constructs that can be the cause of and drive a given behavior. Collaborative metrics address three
different aspects of collaboration: collaboration between the human and the autonomous platform he is
controlling, collaboration among humans that compose the team, and autonomous collaboration among
platforms. These five metric classes have been populated with metrics and measuring techniques from
the existing literature.
Which specific metrics should be used to evaluate a system will depend on many factors, but as a
rule-of-thumb, we propose that at a minimum, one metric from each class should be used to provide a
multi-dimensional assessment of the human-automation team. To determine what the impact on our
research has been by not following such a principled approach, we evaluated recent large-scale
supervisory control experiments conducted in the MIT Humans and Automation Laboratory. The results
show that prior to adapting this metric classification approach, we were fairly consistent in measuring
mission effectiveness and human behavior through such metrics as reaction times and decision
accuracies. However, despite our supervisory control focus, we were remiss in gathering attention
allocation metrics and collaboration metrics, and we often gathered too many correlated metrics that were
redundant and wasteful. This meta-analysis of our experimental shortcomings reflect those in the general
research population in that we tended to gravitate to popular metrics that are relatively easy to gather,
without a clear understanding of exactly what aspect of the systems we were measuring and how the
various metrics informed an overall research question.
Date issued
2008Publisher
MIT Humans and Automation Laboratory
Series/Report no.
HAL Reports;HAL2008-04