A Tree-Based Context Model for Object Recognition
Author(s)
Choi, Myung Jin; Lim, Joseph J.; Torralba, Antonio; Willsky, Alan S.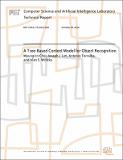
DownloadMIT-CSAIL-TR-2010-050.pdf (13.66Mb)
Other Contributors
Vision
Advisor
Antonio Torralba
Metadata
Show full item recordAbstract
There has been a growing interest in exploiting contextual information in addition to local features to detect and localize multiple object categories in an image. A context model can rule out some unlikely combinations or locations of objects and guide detectors to produce a semantically coherent interpretation of a scene. However, the performance benefit of context models has been limited because most of the previous methods were tested on datasets with only a few object categories, in which most images contain one or two object categories. In this paper, we introduce a new dataset with images that contain many instances of different object categories, and propose an efficient model that captures the contextual information among more than a hundred object categories using a tree structure. Our model incorporates global image features, dependencies between object categories, and outputs of local detectors into one probabilistic framework. We demonstrate that our context model improves object recognition performance and provides a coherent interpretation of a scene, which enables a reliable image querying system by multiple object categories. In addition, our model can be applied to scene understanding tasks that local detectors alone cannot solve, such as detecting objects out of context or querying for the most typical and the least typicalscenes in a dataset.
Date issued
2010-10-29Series/Report no.
MIT-CSAIL-TR-2010-050
Keywords
Object recognition, scene analysis, Markov random fields, structural models, image databases