Distinguishing influence-based contagion from homophily-driven diffusion in dynamic networks
Author(s)
Aral, Sinan; Sundararajan, Arun; Muchnik, Lev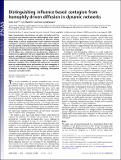
DownloadAral-2009-Distinguishing influ.pdf (1.353Mb)
PUBLISHER_POLICY
Publisher Policy
Article is made available in accordance with the publisher's policy and may be subject to US copyright law. Please refer to the publisher's site for terms of use.
Terms of use
Metadata
Show full item recordAbstract
Node characteristics and behaviors are often correlated with the structure of social networks over time. While evidence of this type of assortative mixing and temporal clustering of behaviors among linked nodes is used to support claims of peer influence and social contagion in networks, homophily may also explain such evidence. Here we develop a dynamic matched sample estimation framework to distinguish influence and homophily effects in dynamic networks, and we apply this framework to a global instant messaging network of 27.4 million users, using data on the day-by-day adoption of a mobile service application and users' longitudinal behavioral, demographic, and geographic data. We find that previous methods overestimate peer influence in product adoption decisions in this network by 300–700%, and that homophily explains >50% of the perceived behavioral contagion. These findings and methods are essential to both our understanding of the mechanisms that drive contagions in networks and our knowledge of how to propagate or combat them in domains as diverse as epidemiology, marketing, development economics, and public health.
Date issued
2009-12Department
Sloan School of ManagementJournal
Proceedings of the National Academy of Sciences of the United States of America
Publisher
National Academy of Sciences
Citation
Aral, Sinan, Lev Muchnik, and Arun Sundararajan. "Distinguishing influence-based contagion from
homophily-driven diffusion in dynamic networks." PNAS December 22, 2009 vol. 106 no. 51. ©2009 by the National Academy of Sciences.
Version: Final published version
ISSN
0027-8424
1091-6490