Tracking employment shocks using mobile phone data
Author(s)
Toole, Jameson Lawrence; Lin, Yu-Ru; Muehlegger, Erich; Shoag, Daniel; Gonzalez, Marta C.; Lazer, David; ... Show more Show less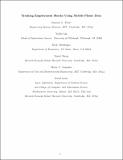
DownloadMain Article (1.565Mb)
OPEN_ACCESS_POLICY
Open Access Policy
Creative Commons Attribution-Noncommercial-Share Alike
Terms of use
Metadata
Show full item recordAbstract
Can data from mobile phones be used to observe economic shocks and their consequences at multiple scales? Here we present novel methods to detect mass layoffs, identify individuals affected by them and predict changes in aggregate unemployment rates using call detail records (CDRs) from mobile phones. Using the closure of a large manufacturing plant as a case study, we first describe a structural break model to correctly detect the date of a mass layoff and estimate its size. We then use a Bayesian classification model to identify affected individuals by observing changes in calling behaviour following the plant's closure. For these affected individuals, we observe significant declines in social behaviour and mobility following job loss. Using the features identified at the micro level, we show that the same changes in these calling behaviours, aggregated at the regional level, can improve forecasts of macro unemployment rates. These methods and results highlight promise of new data resources to measure microeconomic behaviour and improve estimates of critical economic indicators.
Date issued
2015-05Department
Massachusetts Institute of Technology. Department of Civil and Environmental Engineering; Massachusetts Institute of Technology. Engineering Systems DivisionJournal
Journal of The Royal Society Interface
Publisher
Royal Society
Citation
Toole, J. L., Y.-R. Lin, E. Muehlegger, D. Shoag, M. C. Gonzalez, and D. Lazer. “Tracking Employment Shocks Using Mobile Phone Data.” Journal of The Royal Society Interface 12, no. 107 (April 29, 2015): 20150185–20150185.
Version: Author's final manuscript
ISSN
1742-5689
1742-5662