Gaussian Process Decentralized Data Fusion and Active Sensing for Spatiotemporal Traffic Modeling and Prediction in Mobility-on-Demand Systems
Author(s)
Chen, Jie; Low, Kian Hsiang; Yao, Yujian; Jaillet, Patrick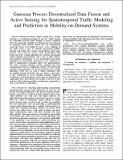
DownloadJaillet_Gaussian process.pdf (567.3Kb)
OPEN_ACCESS_POLICY
Open Access Policy
Creative Commons Attribution-Noncommercial-Share Alike
Terms of use
Metadata
Show full item recordAbstract
Mobility-on-demand (MoD) systems have recently emerged as a promising paradigm of one-way vehicle sharing for sustainable personal urban mobility in densely populated cities. We assume the capability of a MoD system to be enhanced by deploying robotic shared vehicles that can autonomously cruise the streets to be hailed by users. A key challenge of the MoD system is that of real-time, fine-grained mobility demand and traffic flow sensing and prediction. This paper presents novel Gaussian process (GP) decentralized data fusion and active sensing algorithms for real-time, fine-grained traffic modeling and prediction with a fleet of MoD vehicles. The predictive performance of our decentralized data fusion algorithms are theoretically guaranteed to be equivalent to that of sophisticated centralized sparse GP approximations. We derive consensus filtering variants requiring only local communication between neighboring vehicles. We theoretically guarantee the performance of our decentralized active sensing algorithms. When they are used to gather informative data for mobility demand prediction, they can achieve a dual effect of fleet rebalancing to service mobility demands. Empirical evaluation on real-world datasets shows that our algorithms are significantly more time-efficient and scalable in the size of data and fleet while achieving predictive performance comparable to that of state-of-the-art algorithms. Note to Practitioners-Knowing, understanding, and predicting spatiotemporally varying traffic phenomena in real time has become increasingly important to the goal of achieving smooth-flowing, congestion-free traffic in densely populated urban cities, which motivates our work here. This paper addresses the following fundamental problem of data fusion and active sensing: How can a fleet of autonomous robotic vehicles or mobile probes actively cruise a road network to gather and assimilate the most informative data for predicting a spatiotemporally varying traffic phen- menon like a mobility demand pattern or traffic flow? Existing centralized solutions are poorly suited because they suffer from a single point of failure and incur huge communication, space, and time overheads with large data and fleet. This paper proposes novel efficient and scalable decentralized data fusion and active sensing algorithms with theoretical performance guarantees. The practical applicability of our algorithms is not restricted to traffic monitoring [1]-[4]; they can be used in other environmental sensing applications such as mineral prospecting [5], precision agriculture, monitoring of ocean/freshwater phenomena (e.g., plankton bloom) [6]-[9], forest ecosystems, pollution (e.g., oil spill), or contamination. Note that the decentralized data fusion component of our algorithms can also be used for static sensors and passive mobile probes and, interestingly, adapted to parallel implementations to be run on a cluster of machines for achieving efficient and scalable probabilistic prediction (i.e., with predictive uncertainty) with large data. Empirical results show that our algorithms can perform well with two datasets featuring real-world traffic phenomena in the densely-populated urban city of Singapore. A limitation of our algorithms is that the decentralized data fusion components assume independence between multiple traffic phenomena while the decentralized active sensing components only work for a single traffic phenomenon. So, in our future work, we will generalize our algorithms to perform active sensing of multiple traffic phenomena and remove the assumption of independence between them.
Date issued
2015-05Department
Massachusetts Institute of Technology. Department of Electrical Engineering and Computer ScienceJournal
IEEE Transactions on Automation Science and Engineering
Publisher
Institute of Electrical and Electronics Engineers (IEEE)
Citation
Chen, Jie, Kian Hsiang Low, Yujian Yao, and Patrick Jaillet. “Gaussian Process Decentralized Data Fusion and Active Sensing for Spatiotemporal Traffic Modeling and Prediction in Mobility-on-Demand Systems.” IEEE Trans. Automat. Sci. Eng. 12, no. 3 (July 2015): 901–921.
Version: Author's final manuscript
ISSN
1545-5955
1558-3783