Learning Data-Driven Patient Risk Stratification Models for Clostridium difficile
Author(s)
Wiens, Jenna; Campbell, Wayne N.; Franklin, Ella S.; Guttag, John V.; Horvitz, Eric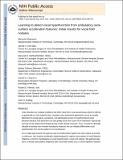
DownloadGuttag_Learning to.pdf (612.2Kb)
PUBLISHER_CC
Publisher with Creative Commons License
Creative Commons Attribution
Terms of use
Metadata
Show full item recordAbstract
Background. Although many risk factors are well known, Clostridium difficile infection (CDI) continues to be a significant problem throughout the world. The purpose of this study was to develop and validate a data-driven, hospital-specific risk stratification procedure for estimating the probability that an inpatient will test positive for C difficile.
Methods. We consider electronic medical record (EMR) data from patients admitted for ≥24 hours to a large urban hospital in the U.S. between April 2011 and April 2013. Predictive models were constructed using L2-regularized logistic regression and data from the first year. The number of observational variables considered varied from a small set of well known risk factors readily available to a physician to over 10 000 variables automatically extracted from the EMR. Each model was evaluated on holdout admission data from the following year. A total of 34 846 admissions with 372 cases of CDI was used to train the model.
Results. Applied to the separate validation set of 34 722 admissions with 355 cases of CDI, the model that made use of the additional EMR data yielded an area under the receiver operating characteristic curve (AUROC) of 0.81 (95% confidence interval [CI], .79–.83), and it significantly outperformed the model that considered only the small set of known clinical risk factors, AUROC of 0.71 (95% CI, .69–.75).
Conclusions. Automated risk stratification of patients based on the contents of their EMRs can be used to accurately identify a high-risk population of patients. The proposed method holds promise for enabling the selective allocation of interventions aimed at reducing the rate of CDI.
Date issued
2014-06Department
Massachusetts Institute of Technology. Department of Electrical Engineering and Computer ScienceJournal
Open Forum Infectious Diseases
Publisher
Oxford University Press
Citation
Wiens, J., W. N. Campbell, E. S. Franklin, J. V. Guttag, and E. Horvitz. “Learning Data-Driven Patient Risk Stratification Models for Clostridium Difficile.” Open Forum Infectious Diseases 1, no. 2 (June 18, 2014): ofu045–ofu045.
Version: Final published version
ISSN
2328-8957