Analytics for an Online Retailer: Demand Forecasting and Price Optimization
Author(s)
Ferreira, Kris Johnson; Lee, Bin Hong Alex; Simchi-Levi, David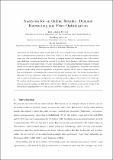
DownloadAnalytics for an Online Retailer - Demand Forecasting and Price Optimization at Rue La La.pdf (1.342Mb)
OPEN_ACCESS_POLICY
Open Access Policy
Creative Commons Attribution-Noncommercial-Share Alike
Terms of use
Metadata
Show full item recordAbstract
We present our work with an online retailer, Rue La La, as an example of how a retailer can use its wealth of data to optimize pricing decisions on a daily basis. Rue La La is in the online fashion sample sales industry, where they offer extremely limited-time discounts on designer apparel and accessories. One of the retailer’s main challenges is pricing and predicting demand for products that it has never sold before, which account for the majority of sales and revenue. To tackle this challenge, we use machine learning techniques to estimate historical lost sales and predict future demand of new products. The nonparametric structure of our demand prediction model, along with the dependence of a product’s demand on the price of competing products, pose new challenges on translating the demand forecasts into a pricing policy. We develop an algorithm to efficiently solve the subsequent multiproduct price optimization that incorporates reference price effects, and we create and implement this algorithm into a pricing decision support tool for Rue La La’s daily use. We conduct a field experiment and find that sales does not decrease because of implementing tool recommended price increases for medium and high price point products. Finally, we estimate an increase in revenue of the test group by approximately 9.7% with an associated 90% confidence interval of [2.3%, 17.8%].
Date issued
2015-11Department
Massachusetts Institute of Technology. Institute for Data, Systems, and Society; Massachusetts Institute of Technology. Department of Civil and Environmental Engineering; Massachusetts Institute of Technology. Engineering Systems Division; Massachusetts Institute of Technology. Operations Research CenterJournal
Manufacturing & Service Operations Management
Publisher
Institute for Operations Research and the Management Sciences (INFORMS)
Citation
Ferreira, Kris Johnson, Bin Hong Alex Lee, and David Simchi-Levi. “Analytics for an Online Retailer: Demand Forecasting and Price Optimization.” M&SOM (November 13, 2015).
Version: Author's final manuscript
ISSN
1523-4614
1526-5498