Scalable Predictive Analysis in Critically Ill Patients Using a Visual Open Data Analysis Platform
Author(s)
Poucke, Sven Van; Zhang, Zhongheng; Schmitz, Martin; Vukicevic, Milan; Laenen, Margot Vander; Deyne, Cathy De; Celi, Leo Anthony G.; ... Show more Show less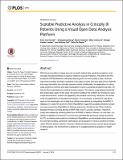
DownloadPoucke-2016-Scalable Predictive.pdf (826.7Kb)
PUBLISHER_CC
Publisher with Creative Commons License
Creative Commons Attribution
Terms of use
Metadata
Show full item recordAbstract
With the accumulation of large amounts of health related data, predictive analytics could stimulate the transformation of reactive medicine towards Predictive, Preventive and Personalized (PPPM) Medicine, ultimately affecting both cost and quality of care. However, high-dimensionality and high-complexity of the data involved, prevents data-driven methods from easy translation into clinically relevant models. Additionally, the application of cutting edge predictive methods and data manipulation require substantial programming skills, limiting its direct exploitation by medical domain experts. This leaves a gap between potential and actual data usage. In this study, the authors address this problem by focusing on open, visual environments, suited to be applied by the medical community. Moreover, we review code free applications of big data technologies. As a showcase, a framework was developed for the meaningful use of data from critical care patients by integrating the MIMIC-II database in a data mining environment (RapidMiner) supporting scalable predictive analytics using visual tools (RapidMiner’s Radoop extension). Guided by the CRoss-Industry Standard Process for Data Mining (CRISP-DM), the ETL process (Extract, Transform, Load) was initiated by retrieving data from the MIMIC-II tables of interest. As use case, correlation of platelet count and ICU survival was quantitatively assessed. Using visual tools for ETL on Hadoop and predictive modeling in RapidMiner, we developed robust processes for automatic building, parameter optimization and evaluation of various predictive models, under different feature selection schemes. Because these processes can be easily adopted in other projects, this environment is attractive for scalable predictive analytics in health research.
Date issued
2016-01Department
Massachusetts Institute of Technology. Institute for Medical Engineering & ScienceJournal
PLOS ONE
Publisher
Public Library of Science
Citation
Poucke, Sven Van, Zhongheng Zhang, Martin Schmitz, Milan Vukicevic, Margot Vander Laenen, Leo Anthony Celi, and Cathy De Deyne. “Scalable Predictive Analysis in Critically Ill Patients Using a Visual Open Data Analysis Platform.” Edited by Tudor Groza. PLoS ONE 11, no. 1 (January 5, 2016): e0145791.
Version: Final published version
ISSN
1932-6203