Enhancing physiologic simulations using supervised learning on coarse mesh solutions
Author(s)
Kolandaivelu, Kumaran; O'Brien, Caroline C.; Shazly, Tarek; Kolachalama, Vijaya Bhasker; Edelman, Elazer R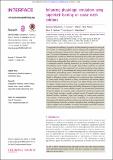
DownloadEdelman_Enhancing physiologic.pdf (1015.Kb)
PUBLISHER_CC
Publisher with Creative Commons License
Creative Commons Attribution
Terms of use
Metadata
Show full item recordAbstract
Computational modelling of physical and biochemical processes has emerged as a means of evaluating medical devices, offering new insights that explain current performance, inform future designs and even enable personalized use. Yet resource limitations force one to compromise with reduced order computational models and idealized assumptions that yield either qualitative descriptions or approximate, quantitative solutions to problems of interest. Considering endovascular drug delivery as an exemplary scenario, we used a supervised machine learning framework to process data generated from low fidelity coarse meshes and predict high fidelity solutions on refined mesh configurations. We considered two models simulating drug delivery to the arterial wall: (i) two-dimensional drug-coated balloons and (ii) three-dimensional drug-eluting stents. Simulations were performed on computational mesh configurations of increasing density. Supervised learners based on Gaussian process modelling were constructed from combinations of coarse mesh setting solutions of drug concentrations and nearest neighbourhood distance information as inputs, and higher fidelity mesh solutions as outputs. These learners were then used as computationally inexpensive surrogates to extend predictions using low fidelity information to higher levels of mesh refinement. The cross-validated, supervised learner-based predictions improved fidelity as compared with computational simulations performed at coarse level meshes—a result consistent across all outputs and computational models considered. Supervised learning on coarse mesh solutions can augment traditional physics-based modelling of complex physiologic phenomena. By obtaining efficient solutions at a fraction of the computational cost, this framework has the potential to transform how modelling approaches can be applied in the evaluation of medical technologies and their real-time administration in an increasingly personalized fashion.
Date issued
2015-02Department
Massachusetts Institute of Technology. Institute for Medical Engineering & ScienceJournal
Journal of The Royal Society Interface
Publisher
Royal Society Publishing
Citation
Kolandaivelu, Kumaran, Caroline C. O’Brien, Tarek Shazly, Elazer R. Edelman, and Vijaya B. Kolachalama. “Enhancing Physiologic Simulations Using Supervised Learning on Coarse Mesh Solutions.” Journal of The Royal Society Interface 12, no. 104 (February 4, 2015): 20141073–20141073.
Version: Final published version
ISSN
1742-5689
1742-5662