Learning Collective Crowd Behaviors with Dynamic Pedestrian-Agents
Author(s)
Zhou, Bolei; Tang, Xiaoou; Wang, Xiaogang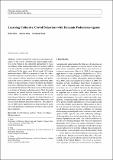
Download11263_2014_735_ReferencePDF.pdf (7.483Mb)
PUBLISHER_POLICY
Publisher Policy
Article is made available in accordance with the publisher's policy and may be subject to US copyright law. Please refer to the publisher's site for terms of use.
Terms of use
Metadata
Show full item recordAbstract
Collective behaviors characterize the intrinsic dynamics of the crowds. Automatically understanding collective crowd behaviors has important applications to video surveillance, traffic management and crowd control, while it is closely related to scientific fields such as statistical physics and biology. In this paper, a new mixture model of dynamic pedestrian-Agents (MDA) is proposed to learn the collective behavior patterns of pedestrians in crowded scenes from video sequences. From agent-based modeling, each pedestrian in the crowd is driven by a dynamic pedestrian-agent, which is a linear dynamic system with initial and termination states reflecting the pedestrian’s belief of the starting point and the destination. The whole crowd is then modeled as a mixture of dynamic pedestrian-agents. Once the model parameters are learned from the trajectories extracted from videos, MDA can simulate the crowd behaviors. It can also infer the past behaviors and predict the future behaviors of pedestrians given their partially observed trajectories, and classify them different pedestrian behaviors. The effectiveness of MDA and its applications are demonstrated by qualitative and quantitative experiments on various video surveillance sequences.
Date issued
2014-06Department
Massachusetts Institute of Technology. Department of Electrical Engineering and Computer ScienceJournal
International Journal of Computer Vision
Publisher
Springer US
Citation
Zhou, Bolei, Xiaoou Tang, and Xiaogang Wang. "Learning Collective Crowd Behaviors with Dynamic Pedestrian-Agents." International Journal of Computer Vision 111:1 (January 2015), pp 50-68.
Version: Author's final manuscript
ISSN
0920-5691
1573-1405