Using informative behavior to increase engagement while learning from human reward
Author(s)
Li, Guangliang; Whiteson, Shimon; Knox, W. Bradley; Hung, Hayley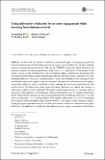
Download10458_2015_Article_9308.pdf (1.905Mb)
PUBLISHER_CC
Publisher with Creative Commons License
Creative Commons Attribution
Terms of use
Metadata
Show full item recordAbstract
In this work, we address a relatively unexplored aspect of designing agents that learn from human reward. We investigate how an agent’s non-task behavior can affect a human trainer’s training and agent learning. We use the TAMER framework, which facilitates the training of agents by human-generated reward signals, i.e., judgements of the quality of the agent’s actions, as the foundation for our investigation. Then, starting from the premise that the interaction between the agent and the trainer should be bi-directional, we propose two new training interfaces to increase a human trainer’s active involvement in the training process and thereby improve the agent’s task performance. One provides information on the agent’s uncertainty which is a metric calculated as data coverage, the other on its performance. Our results from a 51-subject user study show that these interfaces can induce the trainers to train longer and give more feedback. The agent’s performance, however, increases only in response to the addition of performance-oriented information, not by sharing uncertainty levels. These results suggest that the organizational maxim about human behavior, “you get what you measure”—i.e., sharing metrics with people causes them to focus on optimizing those metrics while de-emphasizing other objectives—also applies to the training of agents. Using principle component analysis, we show how trainers in the two conditions train agents differently. In addition, by simulating the influence of the agent’s uncertainty–informative behavior on a human’s training behavior, we show that trainers could be distracted by the agent sharing its uncertainty levels about its actions, giving poor feedback for the sake of reducing the agent’s uncertainty without improving the agent’s performance.
Date issued
2015-08Department
Massachusetts Institute of Technology. Personal Robots GroupJournal
Autonomous Agents and Multi-Agent Systems
Publisher
Springer US
Citation
Li, Guangliang, Shimon Whiteson, W. Bradley Knox, and Hayley Hung. “Using Informative Behavior to Increase Engagement While Learning from Human Reward.” Autonomous Agents and Multi-Agent Systems (August 22, 2015). doi:10.1007/s10458-015-9308-2.
Version: Final published version
ISSN
1387-2532
1573-7454