Compression approaches for the regularized solutions of linear systems from large-scale inverse problems
Author(s)
Voronin, Sergey; Nolet, Guust; Mikesell, T. Dylan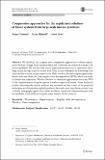
Download13137_2015_Article_73.pdf (4.678Mb)
PUBLISHER_POLICY
Publisher Policy
Article is made available in accordance with the publisher's policy and may be subject to US copyright law. Please refer to the publisher's site for terms of use.
Terms of use
Metadata
Show full item recordAbstract
We introduce and compare new compression approaches to obtain regularized solutions of large linear systems which are commonly encountered in large scale inverse problems. We first describe how to approximate matrix vector operations with a large matrix through a sparser matrix with fewer nonzero elements, by borrowing from ideas used in wavelet image compression. Next, we describe and compare approaches based on the use of the low rank singular value decomposition (SVD), which can result in further size reductions. We describe how to obtain the approximate low rank SVD of the original matrix using the sparser wavelet compressed matrix. Some analytical results concerning the various methods are presented and the results of the proposed techniques are illustrated using both synthetic data and a very large linear system from a seismic tomography application, where we obtain significant compression gains with our methods, while still resolving the main features of the solutions.
Date issued
2015-05Department
Massachusetts Institute of Technology. Department of Earth, Atmospheric, and Planetary SciencesJournal
GEM - International Journal on Geomathematics
Publisher
Springer Berlin Heidelberg
Citation
Voronin, Sergey, Dylan Mikesell, and Guust Nolet. “Compression Approaches for the Regularized Solutions of Linear Systems from Large-Scale Inverse Problems.” Int J Geomath 6, no. 2 (May 19, 2015): 251–294.
Version: Author's final manuscript
ISSN
1869-2672
1869-2680