Maximum likelihood inference in weakly identified dynamic stochastic general equilibrium models
Author(s)
Andrews, Isaiah Smith; Mikusheva, Anna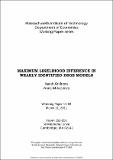
DownloadMikusheva_Maximum likelihood.pdf (437.9Kb)
OPEN_ACCESS_POLICY
Open Access Policy
Creative Commons Attribution-Noncommercial-Share Alike
Terms of use
Metadata
Show full item recordAbstract
This paper examines the issue of weak identification in maximum likelihood, motivated by problems with estimation and inference in a multidimensional dynamic stochastic general equilibrium model. We show that two forms of the classical score (Lagrange multiplier) test for a simple hypothesis concerning the full parameter vector are robust to weak identification. We also suggest a test for a composite hypothesis regarding a subvector of parameters. The suggested subset test is shown to be asymptotically exact when the nuisance parameter is strongly identified. We pay particular attention to the question of how to estimate Fisher information and we make extensive use of martingale theory.
Date issued
2015-03Department
Massachusetts Institute of Technology. Department of EconomicsJournal
Quantitative Economics
Publisher
John Wiley & Sons
Citation
Andrews, Isaiah, and Anna Mikusheva. “Maximum Likelihood Inference in Weakly Identified Dynamic Stochastic General Equilibrium Models.” Quantitative Economics 6, no. 1 (March 2015): 123-152.
Version: Original manuscript
ISSN
17597323