Surrogate and reduced-order modeling: a comparison of approaches for large-scale statistical inverse problems [Chapter 7]
Author(s)
van Bloemen Waanders, B.; Frangos, Michalis; Marzouk, Youssef M; Willcox, Karen E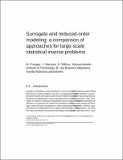
DownloadFrangos2010.pdf (684.1Kb)
OPEN_ACCESS_POLICY
Open Access Policy
Creative Commons Attribution-Noncommercial-Share Alike
Terms of use
Metadata
Show full item recordAbstract
Solution of statistical inverse problems via the frequentist or Bayesian approaches described in earlier chapters can be a computationally intensive endeavor, particularly when faced with large-scale forward models characteristic of many engineering and science applications. High computational cost arises in several ways. First, thousands or millions of forward simulations may be required to evaluate estimators of interest or to characterize a posterior distribution. In the large-scale setting, performing so many forward simulations is often computationally intractable. Second, sampling may be complicated by the large dimensionality of the input space--as when the inputs are fields represented with spatial discretizations of high dimension--and by nonlinear forward dynamics that lead to multimodal, skewed, and/or strongly correlated posteriors. In this chapter, we present an overview of surrogate and reduced order modeling methods that address these computational challenges. For illustration, we consider a Bayesian formulation of the inverse problem. Though some of the methods we review exploit prior information, they largely focus on simplifying or accelerating evaluations of a stochastic model for the data, and thus are also applicable in a frequentist context.
Date issued
2010-01Department
Massachusetts Institute of Technology. Department of Aeronautics and Astronautics; Massachusetts Institute of Technology. Department of Mechanical EngineeringJournal
Computational Methods for Large-Scale Inverse Problems and Quantification of Uncertainty , Biegler et al. (Eds.)
Publisher
John Wiley & Sons
Citation
Frangos, M., Y. Marzouk, K. Willcox, and B. van Bloemen Waanders (2010). Surrogate and reduced-order modeling: a comparison of approaches for large-scale statistical inverse problems. In Lorenz Biegler, George Biros, Omar Ghattas, Matthias Heinkenschloss, David Keyes, Bani Mallick, Youssef Marzouk, Luis Tenorio, Bart van Bloemen Waanders, and Karen Willcox, eds., Large-scale inverse problems and quantification of uncertainty (pp. 123-149) New York: Wiley.
Version: Author's final manuscript
ISBN
9780470697436
9780470685853