Time series modeling of live-cell shape dynamics for image-based phenotypic profiling
Author(s)
Wells, Alan; Gordonov, Simon; Hwang, Mun Kyung; Gertler, Frank; Lauffenburger, Douglas A; Bathe, Mark; ... Show more Show less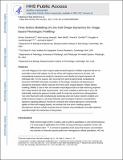
DownloadBathe_Time series modeling.pdf (1.784Mb)
OPEN_ACCESS_POLICY
Open Access Policy
Creative Commons Attribution-Noncommercial-Share Alike
Terms of use
Metadata
Show full item recordAbstract
Live-cell imaging can be used to capture spatio-temporal aspects of cellular responses that are not accessible to fixed-cell imaging. As the use of live-cell imaging continues to increase, new computational procedures are needed to characterize and classify the temporal dynamics of individual cells. For this purpose, here we present the general experimental-computational
framework SAPHIRE (Stochastic Annotation of Phenotypic Individual-cell Responses) to characterize phenotypic cellular responses from time series imaging datasets. Hidden Markov modeling (HMM) is used to infer and annotate morphological state and state-switching properties
from image-derived cell shape measurements. Time series modeling is performed on each cell individually, making the approach broadly useful for analyzing asynchronous cell populations.
Two-color fluorescent cells simultaneously expressing actin and nuclear reporters enabled us to profile temporal changes in cell shape following pharmacological inhibition of cytoskeleton–regulatory signaling pathways. Results are compared with existing approaches conventionally applied to fixed-cell imaging datasets, and indicate that time series modeling captures heterogeneous dynamic cellular responses that can improve drug classification and offer additional important insight into mechanisms of drug action.
Date issued
2015-11Department
Massachusetts Institute of Technology. Department of Biological Engineering; Massachusetts Institute of Technology. Department of Biology; Koch Institute for Integrative Cancer Research at MITJournal
Integrative Biology
Publisher
Royal Society of Chemistry
Citation
Gordonov, Simon et al. “Time Series Modeling of Live-Cell Shape Dynamics for Image-Based Phenotypic Profiling.” Integr. Biol. 8.1 (2016): 73–90.
Version: Author's final manuscript
ISSN
1757-9694
1757-9708