Algorithmic aspects of mean–variance optimization in Markov decision processes
Author(s)
Tsitsiklis, John N; Mannor, Shie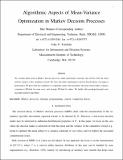
Downloadtsit mv-MDP-EJOR-rev4_SM.pdf (279.7Kb)
PUBLISHER_CC
Publisher with Creative Commons License
Creative Commons Attribution
Terms of use
Metadata
Show full item recordAbstract
We consider finite horizon Markov decision processes under performance measures that involve both the mean and the variance of the cumulative reward. We show that either randomized or history-based policies can improve performance. We prove that the complexity of computing a policy that maximizes the mean reward under a variance constraint is NP-hard for some cases, and strongly NP-hard for others. We finally offer pseudopolynomial exact and approximation algorithms.
Date issued
2013-06Department
Massachusetts Institute of Technology. Department of Electrical Engineering and Computer Science; Massachusetts Institute of Technology. Laboratory for Information and Decision SystemsJournal
European Journal of Operational Research
Publisher
Elsevier
Citation
Mannor, Shie and Tsitsiklis, John N. “Algorithmic Aspects of Mean–variance Optimization in Markov Decision Processes.” European Journal of Operational Research 231, no. 3 (December 2013): 645–653. © 2013 Elsevier B.V.
Version: Author's final manuscript
ISSN
0377-2217