Bayesian model reveals latent atrophy factors with dissociable cognitive trajectories in Alzheimer’s disease
Author(s)
Zhang, Xiuming; Mormino, Elizabeth C.; Sun, Nanbo; Sperling, Reisa A.; Yeo, B. T. Thomas; Sabuncu, Mert R; ... Show more Show less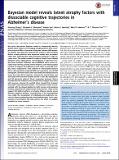
DownloadBayesian model reveals.pdf (10.63Mb)
PUBLISHER_POLICY
Publisher Policy
Article is made available in accordance with the publisher's policy and may be subject to US copyright law. Please refer to the publisher's site for terms of use.
Terms of use
Metadata
Show full item recordAbstract
We used a data-driven Bayesian model to automatically identify distinct latent factors of overlapping atrophy patterns from voxelwise structural MRIs of late-onset Alzheimer’s disease (AD) dementia patients. Our approach estimated the extent to which multiple distinct atrophy patterns were expressed within each participant rather than assuming that each participant expressed a single atrophy factor. The model revealed a temporal atrophy factor (medial temporal cortex, hippocampus, and amygdala), a subcortical atrophy factor (striatum, thalamus, and cerebellum), and a cortical atrophy factor (frontal, parietal, lateral temporal, and lateral occipital cortices). To explore the influence of each factor in early AD, atrophy factor compositions were inferred in beta-amyloid–positive (Aβ+) mild cognitively impaired (MCI) and cognitively normal (CN) participants. All three factors were associated with memory decline across the entire clinical spectrum, whereas the cortical factor was associated with executive function decline in Aβ+ MCI participants and AD dementia patients. Direct comparison between factors revealed that the temporal factor showed the strongest association with memory, whereas the cortical factor showed the strongest association with executive function. The subcortical factor was associated with the slowest decline for both memory and executive function compared with temporal and cortical factors. These results suggest that distinct patterns of atrophy influence decline across different cognitive domains. Quantification of this heterogeneity may enable the computation of individual-level predictions relevant for disease monitoring and customized therapies. Factor compositions of participants and code used in this article are publicly available for future research.
Date issued
2016-10Department
Massachusetts Institute of Technology. Computer Science and Artificial Intelligence LaboratoryJournal
Proceedings of the National Academy of Sciences
Publisher
National Academy of Sciences (U.S.)
Citation
Zhang, Xiuming; Mormino, Elizabeth C.; Sun, Nanbo; Sperling, Reisa A.; Sabuncu, Mert R. and Yeo, B. T. Thomas. “Bayesian Model Reveals Latent Atrophy Factors with Dissociable Cognitive Trajectories in Alzheimer’s Disease.” Proceedings of the National Academy of Sciences 113, no. 42 (October 2016): E6535–E6544. © 2016 National Academy of Sciences
Version: Final published version
ISSN
0027-8424
1091-6490