Discovering charge density functionals and structure-property relationships with PROPhet: A general framework for coupling machine learning and first-principles methods
Author(s)
Kolb, Brian; Lentz, Levi Carl; Kolpak, Alexie M.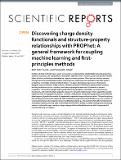
DownloadKolb-2017-Discovering charge density functiona.pdf (1.783Mb)
PUBLISHER_CC
Publisher with Creative Commons License
Creative Commons Attribution
Terms of use
Metadata
Show full item recordAbstract
Modern ab initio methods have rapidly increased our understanding of solid state materials properties, chemical reactions, and the quantum interactions between atoms. However, poor scaling often renders direct ab initio calculations intractable for large or complex systems. There are two obvious avenues through which to remedy this problem: (i) develop new, less expensive methods to calculate system properties, or (ii) make existing methods faster. This paper describes an open source framework designed to pursue both of these avenues. PROPhet (short for PROPerty Prophet) utilizes machine learning techniques to find complex, non-linear mappings between sets of material or system properties. The result is a single code capable of learning analytical potentials, non-linear density functionals, and other structure-property or property-property relationships. These capabilities enable highly accurate mesoscopic simulations, facilitate computation of expensive properties, and enable the development of predictive models for systematic materials design and optimization. This work explores the coupling of machine learning to ab initio methods through means both familiar (e.g., the creation of various potentials and energy functionals) and less familiar (e.g., the creation of density functionals for arbitrary properties), serving both to demonstrate PROPhet’s ability to create exciting post-processing analysis tools and to open the door to improving ab initio methods themselves with these powerful machine learning techniques.
Date issued
2017-04Department
Massachusetts Institute of Technology. Department of Mechanical EngineeringJournal
Scientific Reports
Publisher
Nature Publishing Group
Citation
Kolb, Brian, Levi C. Lentz, and Alexie M. Kolpak. “Discovering Charge Density Functionals and Structure-Property Relationships with PROPhet: A General Framework for Coupling Machine Learning and First-Principles Methods.” Scientific Reports 7.1 (2017): n. pag.
Version: Final published version
ISSN
2045-2322