On the Structure, Covering, and Learning of Poisson Multinomial Distributions
Author(s)
Daskalakis, Konstantinos; Kamath, Gautam Chetan; Tzamos, Christos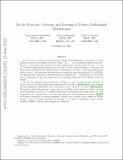
DownloadOn the structure.pdf (525.4Kb)
OPEN_ACCESS_POLICY
Open Access Policy
Creative Commons Attribution-Noncommercial-Share Alike
Terms of use
Metadata
Show full item recordAbstract
An (n, k)-Poisson Multinomial Distribution (PMD) is the distribution of the sum of n independent random vectors supported on the set Bk={e1,...,ek} of standard basis vectors in Rk. We prove a structural characterization of these distributions, showing that, for all ε > 0, any (n, k)-Poisson multinomial random vector is ε-close, in total variation distance, to the sum of a discretized multidimensional Gaussian and an independent (poly(k/ε), k)-Poisson multinomial random vector. Our structural characterization extends the multi-dimensional CLT of Valiant and Valiant, by simultaneously applying to all approximation requirements ε. In particular, it overcomes factors depending on log n and, importantly, the minimum Eigen value of the PMD's covariance matrix. We use our structural characterization to obtain an ε-cover, in total variation distance, of the set of all (n, k)-PMDs, significantly improving the cover size of Daskalakis and Papadimitriou, and obtaining the same qualitative dependence of the cover size on n and ε as the k=2 cover of Daskalakis and Papadimitriou. We further exploit this structure to show that (n, k)-PMDs can be learned to within ε in total variation distance from Õk(1/ε) samples, which is near-optimal in terms of dependence on ε and independent of n. In particular, our result generalizes the single-dimensional result of Daskalakis, Diakonikolas and Servedio for Poisson binomials to arbitrary dimension. Finally, as a corollary of our results on PMDs, we give a Õk(1/ε2) sample algorithm for learning (n, k)-sums of independent integer random variables (SIIRVs), which is near-optimal for constant k.
Date issued
2015-10Department
Massachusetts Institute of Technology. Computer Science and Artificial Intelligence Laboratory; Massachusetts Institute of Technology. Department of Electrical Engineering and Computer ScienceJournal
2015 IEEE 56th Annual Symposium on Foundations of Computer Science
Publisher
Institute of Electrical and Electronics Engineers (IEEE)
Citation
Daskalakis, Constantinos, Gautam Kamath, and Christos Tzamos. “On the Structure, Covering, and Learning of Poisson Multinomial Distributions.” 2015 IEEE 56th Annual Symposium on Foundations of Computer Science (October 2015).
Version: Author's final manuscript
ISBN
978-1-4673-8191-8