Perceptual multistability as Markov Chain Monte Carlo inference
Author(s)
Gershman, Samuel J.; Vul, Edward; Tenenbaum, Joshua B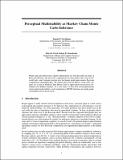
Download3711-perceptual-multistability-as-markov-chain-monte-carlo-inference.pdf (972.8Kb)
PUBLISHER_POLICY
Publisher Policy
Article is made available in accordance with the publisher's policy and may be subject to US copyright law. Please refer to the publisher's site for terms of use.
Terms of use
Metadata
Show full item recordAbstract
While many perceptual and cognitive phenomena are well described in terms of Bayesian inference, the necessary computations are intractable at the scale of real-world tasks, and it remains unclear how the human mind approximates Bayesian computations algorithmically. We explore the proposal that for some tasks, humans use a form of Markov Chain Monte Carlo to approximate the posterior distribution over hidden variables. As a case study, we show how several phenomena of perceptual multistability can be explained as MCMC inference in simple graphical models for low-level vision.
Date issued
2009Department
Massachusetts Institute of Technology. Department of Brain and Cognitive SciencesJournal
Advances in Neural Information Processing Systems (NIPS)
Publisher
Neural Information Processing Systems Foundation
Citation
Gershman, Samuel J. et al. "Perceptual multistability as Markov Chain Monte Carlo inference." Advances in Neural Information Processing Systems (NIPS), December 2009, Vancouver, B.C., Canada, Neural Information Processing Systems Foundation, 2009 © 2009 Neural Information Processing Systems Foundation
Version: Final published version
ISSN
1049-5258