Beyond Boolean logic: exploring representation languages for learning complex concepts
Author(s)
Piantadosi, Steven Thomas; Tenenbaum, Joshua B; Goodman, Noah Daniel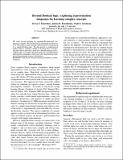
DownloadPiantadosiTenenbaumGoodman2010-CogsciFINAL.pdf (260.5Kb)
OPEN_ACCESS_POLICY
Open Access Policy
Creative Commons Attribution-Noncommercial-Share Alike
Terms of use
Metadata
Show full item recordAbstract
We study concept learning for semantically-motivated, set-theoretic concepts. We first present an experiment in which we show that subjects learn concepts which cannot be represented by a simple Boolean logic. We then present a computational
model which is similarly capable of learning these concepts,and show that it provides a good fit to human learning curves. Additionally, we compare the performance of several potential representation languages which are richer than Boolean logic
in predicting human response distributions.
Keywords: Rule-based concept learning; probabilistic model;semantics.
Date issued
2010-08Department
Massachusetts Institute of Technology. Department of Brain and Cognitive SciencesJournal
32nd Annual Meeting of the Cognitive Science Society 2010
Publisher
Cognitive Science Society
Citation
Piantadosi, Steven T. et al. "Beyond Boolean logic: exploring representation languages for learning complex concepts." 32nd Annual Meeting of the Cognitive Science Society 2010, August 11-14 2010, Portland, Oregon, USA, Cognitive Science Society, August 2010 © 2010 Cognitive Science Society
Version: Author's final manuscript
ISBN
978-1-61738-890-3