An efficient algorithm for information decomposition and extraction
Author(s)
Makur, Anuran; Kozynski Waserman, Fabian Ariel; Huang, Shao-Lun; Zheng, Lizhong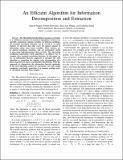
DownloadZheng_An efficient.pdf (318.7Kb)
OPEN_ACCESS_POLICY
Open Access Policy
Creative Commons Attribution-Noncommercial-Share Alike
Terms of use
Metadata
Show full item recordAbstract
The Hirschfeld-Gebelein-Rényi maximal correlation is a well-known measure of statistical dependence between two (possibly categorical) random variables. In inference problems, the maximal correlation functions can be viewed as so called features of observed data that carry the largest amount of information about some latent variables. These features are in general non-linear functions, and are particularly useful in processing high-dimensional observed data. The alternating conditional expectations (ACE) algorithm is an efficient way to compute these maximal correlation functions. In this paper, we use an information theoretic approach to interpret the ACE algorithm as computing the singular value decomposition of a linear map between spaces of probability distributions. With this approach, we demonstrate the information theoretic optimality of the ACE algorithm, analyze its convergence rate and sample complexity, and finally, generalize it to compute multiple pairs of correlation functions from samples.
Date issued
2015-09Department
Massachusetts Institute of Technology. Department of Electrical Engineering and Computer ScienceJournal
2015 53rd Annual Allerton Conference on Communication, Control, and Computing (Allerton)
Publisher
Institute of Electrical and Electronics Engineers (IEEE)
Citation
Makur, Anuran et al.. “An Efficient Algorithm for Information Decomposition and Extraction.” 2015 53rd Annual Allerton Conference on Communication, Control, and Computing (Allerton) 29 September - 2 October, 2015, Monticello, Illinois, 2015.
Version: Author's final manuscript
ISBN
978-1-5090-1824-6