Bayesian optimization with exponential convergence
Author(s)
Kawaguchi, Kenji; Kaelbling, Leslie P; Lozano-Perez, Tomas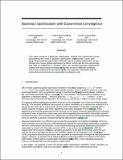
DownloadLozano-Perez_Bayesian optimization.pdf (1.002Mb)
PUBLISHER_POLICY
Publisher Policy
Article is made available in accordance with the publisher's policy and may be subject to US copyright law. Please refer to the publisher's site for terms of use.
Terms of use
Metadata
Show full item recordAbstract
This paper presents a Bayesian optimization method with exponential convergence without the need of auxiliary optimization and without the delta-cover sampling. Most Bayesian optimization methods require auxiliary optimization: an additional non-convex global optimization problem, which can be time-consuming and hard to implement in practice. Also, the existing Bayesian optimization method with exponential convergence requires access to the delta-cover sampling, which was considered to be impractical. Our approach eliminates both requirements and achieves an exponential convergence rate.
Date issued
2015-12Department
Massachusetts Institute of Technology. Department of Electrical Engineering and Computer ScienceJournal
Advances in Neural Information Processing Systems 28 (NIPS 2015)
Publisher
Neural Information Processing Systems Foundation,
Citation
Kawaguchi, Kenji, Leslie Pack Kaelbling, and Tomás Lozano-Pérez. "Bayesian Optimization with Exponential Convergence." Advances in Neural Information Processing Systems 28 (NIPS 2015), 7-12 December, 2015, Montreal, Canada, Neural Information Processing Systems Foundation, 2015. © 2015 Neural Information Processing Systems Foundation
Version: Final published version