Learning a Health Knowledge Graph from Electronic Medical Records
Author(s)
Rotmensch, Maya; Halpern, Yoni; Tlimat, Abdulhakim; Horng, Steven; Sontag, David Alexander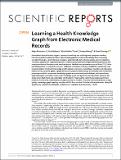
Downloads41598-017-05778-z.pdf (2.766Mb)
PUBLISHER_POLICY
Publisher Policy
Article is made available in accordance with the publisher's policy and may be subject to US copyright law. Please refer to the publisher's site for terms of use.
Terms of use
Metadata
Show full item recordAbstract
Demand for clinical decision support systems in medicine and self-diagnostic symptom checkers has substantially increased in recent years. Existing platforms rely on knowledge bases manually compiled through a labor-intensive process or automatically derived using simple pairwise statistics. This study explored an automated process to learn high quality knowledge bases linking diseases and symptoms directly from electronic medical records. Medical concepts were extracted from 273,174 de-identified patient records and maximum likelihood estimation of three probabilistic models was used to automatically construct knowledge graphs: logistic regression, naive Bayes classifier and a Bayesian network using noisy OR gates. A graph of disease-symptom relationships was elicited from the learned parameters and the constructed knowledge graphs were evaluated and validated, with permission, against Google’s manually-constructed knowledge graph and against expert physician opinions. Our study shows that direct and automated construction of high quality health knowledge graphs from medical records using rudimentary concept extraction is feasible. The noisy OR model produces a high quality knowledge graph reaching precision of 0.85 for a recall of 0.6 in the clinical evaluation. Noisy OR significantly outperforms all tested models across evaluation frameworks (p < 0.01).
Date issued
2017-07Department
Institute for Medical Engineering and Science; Massachusetts Institute of Technology. Computer Science and Artificial Intelligence Laboratory; Massachusetts Institute of Technology. Department of Electrical Engineering and Computer ScienceJournal
Scientific Reports
Publisher
Springer Nature
Citation
Rotmensch, Maya, Yoni Halpern, Abdulhakim Tlimat, Steven Horng, and David Sontag. “Learning a Health Knowledge Graph from Electronic Medical Records.” Scientific Reports 7, no. 1 (July 20, 2017).
Version: Final published version
ISSN
2045-2322