Gaussian approximation of suprema of empirical processes
Author(s)
Chetverikov, Denis; Kato, Kengo; Chernozhukov, Victor V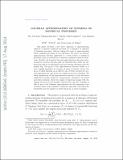
Download1212.6885.pdf (545.2Kb)
OPEN_ACCESS_POLICY
Open Access Policy
Creative Commons Attribution-Noncommercial-Share Alike
Terms of use
Metadata
Show full item recordAbstract
This paper develops a new direct approach to approximating suprema of general empirical processes by a sequence of suprema of Gaussian processes, without taking the route of approximating whole empirical processes in the sup-norm. We prove an abstract approximation theorem applicable to a wide variety of statistical problems, such as construction of uniform confidence bands for functions. Notably, the bound in the main approximation theorem is nonasymptotic and the theorem allows for functions that index the empirical process to be unbounded and have entropy divergent with the sample size. The proof of the approximation theorem builds on a new coupling inequality for maxima of sums of random vectors, the proof of which depends on an effective use of Stein's method for normal approximation, and some new empirical process techniques. We study applications of this approximation theorem to local and series empirical processes arising in nonparametric estimation via kernel and series methods, where the classes of functions change with the sample size and are non-Donsker. Importantly, our new technique is able to prove the Gaussian approximation for the supremum type statistics under weak regularity conditions, especially concerning the bandwidth and the number of series functions, in those examples. Keywords: coupling; empirical process; Gaussian approximation; kernel estimation; local empirical process; series estimation; supremum
Date issued
2014-08Department
Massachusetts Institute of Technology. Department of EconomicsJournal
The Annals of Statistics
Publisher
Institute of Mathematical Statistics
Citation
Chernozhukov, Victor et al. “Gaussian Approximation of Suprema of Empirical Processes.” The Annals of Statistics 42, 4 (August 2014): 1564–1597 © 2014 Institute of Mathematical Statistics
Version: Author's final manuscript
ISSN
0090-5364