Bayesian Inverse Problems with L[subscript 1] Priors: A Randomize-Then-Optimize Approach
Author(s)
Bardsley, Johnathan M.; Solonen, Antti; Cui, Tiangang; Wang, Zheng; Marzouk, Youssef M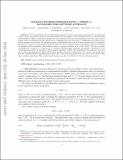
Download1607.01904.pdf (1.231Mb)
OPEN_ACCESS_POLICY
Open Access Policy
Creative Commons Attribution-Noncommercial-Share Alike
Terms of use
Metadata
Show full item recordAbstract
Prior distributions for Bayesian inference that rely on the L[subscript 1]-norm of the parameters are of considerable interest, in part because they promote parameter fields with less regularity than Gaussian priors (e.g., discontinuities and blockiness). These L[subscript 1]-type priors include the total variation (TV) prior and the Besov space B[subscript 1,1][superscript s] prior, and in general yield non-Gaussian posterior distributions. Sampling from these posteriors is challenging, particularly in the inverse problem setting where the parameter space is high-dimensional and the forward problem may be nonlinear. This paper extends the randomize-then-optimize (RTO) method, an optimization-based sampling algorithm developed for Bayesian inverse problems with Gaussian priors, to inverse problems with L[subscript 1]-type priors. We use a variable transformation to convert an L[subscript 1]-type prior to a standard Gaussian prior, such that the posterior distribution of the transformed parameters is amenable to Metropolized sampling via RTO. We demonstrate this approach on several deconvolution problems and an elliptic PDE inverse problem, using TV or Besov space B[subscript 1,1][superscript s] priors. Our results show that the transformed RTO algorithm characterizes the correct posterior distribution and can be more efficient than other sampling algorithms. The variable transformation can also be extended to other non-Gaussian priors. (An erratum is attached.)
Date issued
2017-01Department
Massachusetts Institute of Technology. Department of Aeronautics and AstronauticsJournal
SIAM Journal on Scientific Computing
Publisher
Society for Industrial & Applied Mathematics (SIAM)
Citation
Wang, Zheng, Johnathan M. Bardsley, Antti Solonen, Tiangang Cui, and Youssef M. Marzouk. “Bayesian Inverse Problems with L[subscript 1] Priors: A Randomize-Then-Optimize Approach.” SIAM Journal on Scientific Computing 39, 5 (January 2017): S140–S166 © 2017 Society for Industrial and Applied Mathematics
Version: Author's final manuscript
ISSN
1064-8275
1095-7197