RCK: accurate and efficient inference of sequence- and structure-based protein–RNA binding models from RNAcompete data
Author(s)
Orenstein, Yaron; Wang, Yuhao; Berger Leighton, Bonnie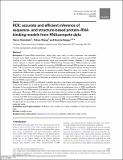
Downloadbtw259.pdf (1016.Kb)
PUBLISHER_CC
Publisher with Creative Commons License
Creative Commons Attribution
Terms of use
Metadata
Show full item recordAbstract
Motivation: Protein-RNA interactions, which play vital roles in many processes, are mediated through both RNA sequence and structure. CLIP-based methods, which measure protein-RNA binding in vivo, suffer from experimental noise and systematic biases, whereas in vitro experiments capture a clearer signal of protein RNA-binding. Among them, RNAcompete provides binding affinities of a specific protein to more than 240 000 unstructured RNA probes in one experiment. The computational challenge is to infer RNA structure- and sequence-based binding models from these data. The state-of-the-art in sequence models, Deepbind, does not model structural preferences. RNAcontext models both sequence and structure preferences, but is outperformed by GraphProt. Unfortunately, GraphProt cannot detect structural preferences from RNAcompete data due to the unstructured nature of the data, as noted by its developers, nor can it be tractably run on the full RNACompete dataset. Results: We develop RCK, an efficient, scalable algorithm that infers both sequence and structure preferences based on a new k-mer based model. Remarkably, even though RNAcompete data is designed to be unstructured, RCK can still learn structural preferences from it. RCK significantly outperforms both RNAcontext and Deepbind in in vitro binding prediction for 244 RNAcompete experiments. Moreover, RCK is also faster and uses less memory, which enables scalability. While currently on par with existing methods in in vivo binding prediction on a small scale test, we demonstrate that RCK will increasingly benefit from experimentally measured RNA structure profiles as compared to computationally predicted ones. By running RCK on the entire RNAcompete dataset, we generate and provide as a resource a set of protein-RNA structure-based models on an unprecedented scale.
Date issued
2016-06Department
Massachusetts Institute of Technology. Computer Science and Artificial Intelligence Laboratory; Massachusetts Institute of Technology. Department of Electrical Engineering and Computer Science; Massachusetts Institute of Technology. Department of MathematicsJournal
Bioinformatics
Publisher
Oxford University Press (OUP)
Citation
Orenstein, Yaron, et al. “RCK: Accurate and Efficient Inference of Sequence- and Structure-Based Protein–RNA Binding Models from RNAcompete Data.” Bioinformatics, vol. 32, no. 12, June 2016, pp. i351–59.
Version: Final published version
ISSN
1367-4803
1460-2059