Collaborative planning with encoding of users' high-level strategies
Author(s)
Banks, Christopher J.; Kim, Joseph; Shah, Julie A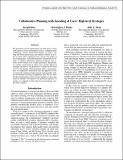
DownloadKim_AAAI_2017_preprint.pdf (1.948Mb)
OPEN_ACCESS_POLICY
Open Access Policy
Creative Commons Attribution-Noncommercial-Share Alike
Terms of use
Metadata
Show full item recordAbstract
© Copyright 2017, Association for the Advancement of Artificial Intelligence (www.aaai.org). All rights reserved. The generation of near-optimal plans for multi-agent systems with numerical states and temporal actions is computationally challenging. Current off-the-shelf planners can take a very long time before generating a near-optimal solution. In an effort to reduce plan computation time, increase the quality of the resulting plans, and make them more interpretable by humans, we explore collaborative planning techniques that actively involve human users in plan generation. Specifically, we explore a framework in which users provide high-level strategies encoded as soft preferences to guide the low-level search of the planner. Through human subject experimentation, we empirically demonstrate that this approach results in statistically significant improvements to plan quality, without substantially increasing computation time. We also show that the resulting plans achieve greater similarity to those generated by humans with regard to the produced sequences of actions, as compared to plans that do not incorporate userprovided strategies.
Date issued
2017-02Department
Massachusetts Institute of Technology. Department of Aeronautics and AstronauticsJournal
Proceedings of the Thirty-First AAAI Conference on Artificial Intelligence (AAAI-17)
Publisher
Association for the Advancement of Artificial Intelligence
Citation
Kim, Joseph, Christopher J. Banks and Julie A. Shah. "Collaborative planning with encoding of users' high-level strategies." In Proceedings of the Thirty-First AAAI Conference on Artificial Intelligence (AAAI-17), San Francisco, California, 4-9 February. Palo Alto, California, Association for the Advancement of Artificial Intelligence 2017, pp. 955-961.
Version: Author's final manuscript
ISBN
9781577357810