Sparse sensing for resource-constrained depth reconstruction
Author(s)
Ma, Fangchang; Carlone, Luca; Ayaz, Ulas; Karaman, Sertac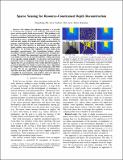
DownloadMa.Carlone.IROS16.pdf (1.812Mb)
OPEN_ACCESS_POLICY
Open Access Policy
Creative Commons Attribution-Noncommercial-Share Alike
Terms of use
Metadata
Show full item recordAbstract
We address the following question: is it possible to reconstruct the geometry of an unknown environment using sparse and incomplete depth measurements? This problem is relevant for a resource-constrained robot that has to navigate and map an environment, but does not have enough on-board power or payload to carry a traditional depth sensor (e.g., a 3D lidar) and can only acquire few (point-wise) depth measurements. In general, reconstruction from incomplete data is not possible, but when the robot operates in man-made environments, the depth exhibits some regularity (e.g., many planar surfaces with few edges); we leverage this regularity to infer depth from incomplete measurements. Our formulation bridges robotic perception with the compressive sensing literature in signal processing. We exploit this connection to provide formal results on exact depth recovery in 2D and 3D problems. Taking advantage of our specific sensing modality, we also prove novel and more powerful results to completely characterize the geometry of the signals that we can reconstruct. Our results directly translate to practical algorithms for depth reconstruction; these algorithms are simple (they reduce to solving a linear program), and robust to noise. We test our algorithms on real and simulated data, and show that they enable accurate depth reconstruction from a handful of measurements, and perform well even when the assumption of structured environment is violated.
Date issued
2016-12Department
Massachusetts Institute of Technology. Department of Aeronautics and Astronautics; Massachusetts Institute of Technology. Department of Electrical Engineering and Computer Science; Massachusetts Institute of Technology. Department of Mechanical Engineering; Massachusetts Institute of Technology. Laboratory for Information and Decision SystemsJournal
2016 IEEE/RSJ International Conference on Intelligent Robots and Systems (IROS)
Publisher
Institute of Electrical and Electronics Engineers (IEEE)
Citation
Ma, Fangchang, Luca Carlone, Ulas Ayaz, and Sertac Karaman. “Sparse Sensing for Resource-Constrained Depth Reconstruction.” 2016 IEEE/RSJ International Conference on Intelligent Robots and Systems (IROS) (October 2016).
Version: Author's final manuscript
ISBN
978-1-5090-3762-9