Inherent limitations of hybrid transactional memory
Author(s)
Alistarh, Dan; Kuznetsov, Petr; Ravi, Srivatsan; Kopinsky, Justin; Shavit, Nir N.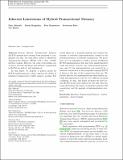
Download446_2017_305_ReferencePDF.pdf (358.4Kb)
OPEN_ACCESS_POLICY
Open Access Policy
Creative Commons Attribution-Noncommercial-Share Alike
Terms of use
Metadata
Show full item recordAbstract
Several hybrid transactional memory (HyTM) schemes have recently been proposed to complement the fast, but best-effort nature of hardware transactional memory with a slow, reliable software backup. However, the costs of providing concurrency between hardware and software transactions in HyTM are still not well understood. In this paper, we propose a general model for HyTM implementations, which captures the ability of hardware transactions to buffer memory accesses. The model allows us to formally quantify and analyze the amount of overhead (instrumentation) caused by the potential presence of software transactions. We prove that (1) it is impossible to build a strictly serializable HyTM implementation that has both uninstrumented reads and writes, even for very weak progress guarantees, and (2) the instrumentation cost incurred by a hardware transaction in any progressive opaque HyTM is linear in the size of the transaction’s data set. We further describe two implementations which exhibit optimal instrumentation costs for two different progress conditions. In sum, this paper proposes the first formal HyTM model and captures for the first time the trade-off between the degree of hardware-software TM concurrency and the amount of instrumentation overhead. Keywords: hardware transactional memory, Instrumentation, Lower bounds
Date issued
2017-06Department
Massachusetts Institute of Technology. Computer Science and Artificial Intelligence Laboratory; Massachusetts Institute of Technology. Department of Electrical Engineering and Computer ScienceJournal
Distributed Computing
Publisher
Springer Berlin Heidelberg
Citation
Alistarh, Dan, et al. “Inherent Limitations of Hybrid Transactional Memory.” Distributed Computing, vol. 31, no. 3, June 2018, pp. 167–85.
Version: Author's final manuscript
ISSN
0178-2770
1432-0452