Efficient NP Tests for Anomaly Detection Over Birth-Death Type DTMCs
Author(s)
Ozkan, Huseyin; Ozkan, Fatih; Delibalta, Ibrahim; Kozat, Suleyman S.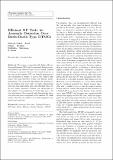
Download11265_2016_1147_ReferencePDF.pdf (392.0Kb)
PUBLISHER_POLICY
Publisher Policy
Article is made available in accordance with the publisher's policy and may be subject to US copyright law. Please refer to the publisher's site for terms of use.
Terms of use
Metadata
Show full item recordAbstract
We propose computationally highly efficient Neyman-Pearson (NP) tests for anomaly detection over birth-death type discrete time Markov chains. Instead of relying on extensive Monte Carlo simulations (as in the case of the baseline NP), we directly approximate the log-likelihood density to match the desired false alarm rate; and therefore obtain our efficient implementations. The proposed algorithms are appropriate for processing large scale data in online applications with real time false alarm rate controllability. Since we do not require parameter tuning, our algorithms are also adaptive to non-stationarity in the data source. In our experiments, the proposed tests demonstrate superior detection power compared to the baseline NP while nearly achieving the desired rates with negligible computational resources. Keywords: Anomaly detection, Neyman pearson, NP, False alarm, Efficient Online, Markov DTMC
Date issued
2016-06Department
Massachusetts Institute of Technology. Department of Brain and Cognitive SciencesJournal
Journal of Signal Processing Systems
Publisher
Springer US
Citation
Ozkan, Huseyin, et al. “Efficient NP Tests for Anomaly Detection Over Birth-Death Type DTMCs.” Journal of Signal Processing Systems, vol. 90, no. 2, Feb. 2018, pp. 175–84.
Version: Author's final manuscript
ISSN
1939-8018
1939-8115