Network inference from consensus dynamics
Author(s)
Segarra, Santiago M; Schaub, Michael T; Jadbabaie-Moghadam, Ali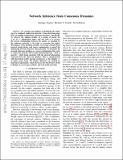
Download1708.05329.pdf (1.644Mb)
OPEN_ACCESS_POLICY
Open Access Policy
Creative Commons Attribution-Noncommercial-Share Alike
Terms of use
Metadata
Show full item recordAbstract
We consider the problem of identifying the topology of a weighted, undirected network G from observing snapshots of multiple independent consensus dynamics. Specifically, we observe the opinion profiles of a group of agents for a set of M independent topics and our goal is to recover the precise relationships between the agents, as specified by the unknown network G. In order to overcome the under-determinacy of the problem at hand, we leverage concepts from spectral graph theory and convex optimization to unveil the underlying network structure. More precisely, we formulate the network inference problem as a convex optimization that seeks to endow the network with certain desired properties - such as sparsity - while being consistent with the spectral information extracted from the observed opinions. This is complemented with theoretical results proving consistency as the number M of topics grows large. We further illustrate our method by numerical experiments, which showcase the effectiveness of the technique in recovering synthetic and real-world networks.
Date issued
2018-01Department
Massachusetts Institute of Technology. Institute for Data, Systems, and SocietyJournal
2017 IEEE 56th Annual Conference on Decision and Control (CDC)
Publisher
Institute of Electrical and Electronics Engineers (IEEE)
Citation
Segarra, Santiago, et al. “Network Inference from Consensus Dynamics.” 2017 IEEE 56th Annual Conference on Decision and Control (CDC), 12-15 December, 2017, Melbourne, Australia, IEEE, 2017, pp. 3212–17.
Version: Author's final manuscript
ISBN
978-1-5090-2873-3