Technical Note—Dynamic Pricing and Demand Learning with Limited Price Experimentation
Author(s)
Cheung, Wang Chi; Simchi-Levi, David; Wang, He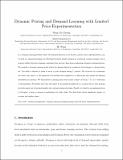
DownloadPricing_v4_a.pdf (557.6Kb)
OPEN_ACCESS_POLICY
Open Access Policy
Creative Commons Attribution-Noncommercial-Share Alike
Terms of use
Metadata
Show full item recordAbstract
In a dynamic pricing problem where the demand function is not known a priori, price experimentation can be used as a demand learning tool. Existing literature usually assumes no constraint on price changes, but in practice, sellers often face business constraints that prevent them from conducting extensive experimentation. We consider a dynamic pricing model where the demand function is unknown but belongs to a known finite set. The seller is allowed to make at most m price changes during T periods. The objective is to minimize the worst-case regret—i.e., the expected total revenue loss compared with a clairvoyant who knows the demand distribution in advance. We demonstrate a pricing policy that incurs a regret of O(log(m)T), or m iterations of the logarithm. Furthermore, we describe an implementation of this pricing policy at Groupon, a large e-commerce marketplace for daily deals. The field study shows significant impact on revenue and bookings.
Date issued
2017-11Department
Massachusetts Institute of Technology. Department of Civil and Environmental Engineering; Massachusetts Institute of Technology. Institute for Data, Systems, and Society; Massachusetts Institute of Technology. Operations Research CenterJournal
Operations Research
Publisher
Institute for Operations Research and the Management Sciences (INFORMS)
Citation
Cheung, Wang Chi, et al. “Technical Note—Dynamic Pricing and Demand Learning with Limited Price Experimentation.” Operations Research, vol. 65, no. 6, Dec. 2017, pp. 1722–31.
Version: Original manuscript
ISSN
0030-364X
1526-5463