A Geometric Approach to Dynamical Model Order Reduction
Author(s)
Feppon, Florian Jeremy; Lermusiaux, Pierre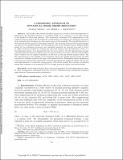
Download16m1095202.pdf (2.110Mb)
PUBLISHER_POLICY
Publisher Policy
Article is made available in accordance with the publisher's policy and may be subject to US copyright law. Please refer to the publisher's site for terms of use.
Terms of use
Metadata
Show full item recordAbstract
Any model order reduced dynamical system that evolves a modal decomposition to approximate the discretized solution of a stochastic PDE can be related to a vector field tangent to the manifold of fixed rank matrices. The dynamically orthogonal (DO) approximation is the canonical reduced-order model for which the corresponding vector field is the orthogonal projection of the original system dynamics onto the tangent spaces of this manifold. The embedded geometry of the fixed rank matrix manifold is thoroughly analyzed. The curvature of the manifold is characterized and related to the smallest singular value through the study of the Weingarten map. Differentiability results for the orthogonal projection onto embedded manifolds are reviewed and used to derive an explicit dynamical system for tracking the truncated singular value decomposition (SVD) of a time-dependent matrix. It is demonstrated that the error made by the DO approximation remains controlled under the minimal condition that the original solution stays close to the low rank manifold, which translates into an explicit dependence of this error on the gap between singular values. The DO approximation is also justified as the dynamical system that applies instantaneously the SVD truncation to optimally constrain the rank of the reduced solution. Riemannian matrix optimization is investigated in this extrinsic framework to provide algorithms that adaptively update the best low rank approximation of a smoothly varying matrix. The related gradient flow provides a dynamical system that converges to the truncated SVD of an input matrix for almost every initial datum. Key words. model order reduction, fixed rank matrix manifold, low rank approximation, singular value decomposition, orthogonal projection, curvature, Weingarten map, dynamically orthogonal approximation, Riemannian matrix optimization
Date issued
2018-01Department
Massachusetts Institute of Technology. Department of Mechanical Engineering; Massachusetts Institute of Technology. Computation for Design and Optimization ProgramJournal
SIAM Journal on Matrix Analysis and Applications
Publisher
Society for Industrial & Applied Mathematics (SIAM)
Citation
Feppon, Florian, and Pierre F. J. Lermusiaux. “A Geometric Approach to Dynamical Model Order Reduction.” SIAM Journal on Matrix Analysis and Applications 39, no. 1 (January 2018): 510–538. © 2018 Society for Industrial and Applied Mathematics.
Version: Final published version
ISSN
0895-4798
1095-7162