What Can Machines Learn, and What Does It Mean for Occupations and the Economy?
Author(s)
Brynjolfsson, Erik; Mitchell, Tom; Rock, Daniel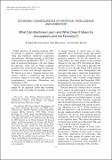
Downloadpandp.20181019.pdf (636.0Kb)
PUBLISHER_POLICY
Publisher Policy
Article is made available in accordance with the publisher's policy and may be subject to US copyright law. Please refer to the publisher's site for terms of use.
Terms of use
Metadata
Show full item recordAbstract
Advances in machine learning (ML) are poised to transform numerous occupations and industries. This raises the question of which tasks will be most affected by ML. We apply the rubric evaluating task potential for ML in Brynjolfsson and Mitchell (2017) to build measures of "Suitability for Machine Learning" (SML) and apply it to 18,156 tasks in O*NET. We find that (i) ML affects different occupations than earlier automation waves; (ii) most occupations include at least some SML tasks; (iii) few occupations are fully automatable using ML; and (iv) realizing the potential of ML usually requires redesign of job task content.
Date issued
2018-05Department
Sloan School of ManagementJournal
AEA Papers and Proceedings
Publisher
American Economic Association
Citation
Brynjolfsson, Erik, Tom Mitchell, and Daniel Rock. “What Can Machines Learn, and What Does It Mean for Occupations and the Economy?” AEA Papers and Proceedings 108 (2018): 43–47.
Version: Final published version
ISSN
2574-0768