Dynamic Pricing through Data Sampling
Author(s)
Lobel, Ruben; Cohen, Maxime; Perakis, Georgia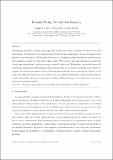
Downloada613003c22f2a51925bf0442fdab581ef87a.pdf (416.0Kb)
OPEN_ACCESS_POLICY
Open Access Policy
Creative Commons Attribution-Noncommercial-Share Alike
Terms of use
Metadata
Show full item recordAbstract
We study a dynamic pricing problem, where a firm offers a product to be sold over a fixed time horizon. The firm has a given initial inventory level, but there is uncertainty about the demand for the product in each time period. The objective of the firm is to determine a dynamic pricing strategy that maximizes revenue throughout the entire selling season. We develop a tractable optimization model that directly uses demand data, therefore creating a practical decision tool. We show computationally that regret-based objectives can perform well when compared to average revenue maximization and to a Bayesian approach. The modeling approach proposed in this study could be particularly useful for risk-averse managers with limited access to historical data or information about the true demand distribution. Finally, we provide theoretical performance guarantees for this sampling-based solution.
Date issued
2018-02Department
Massachusetts Institute of Technology. Operations Research Center; Sloan School of ManagementJournal
Production and Operations Management
Publisher
Wiley
Citation
Cohen, Maxime C., Ruben Lobel, and Georgia Perakis. “Dynamic Pricing through Data Sampling.” Production and Operations Management 27, no. 6 (March 1, 2018): 1074–1088.
Version: Original manuscript
ISSN
1059-1478