Activity-Based Human Mobility Patterns Inferred from Mobile Phone Data: A Case Study of Singapore
Author(s)
Jiang, Shan; Ferreira Jr, Joseph; Gonzalez, Marta C.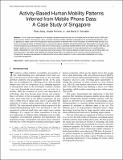
Downloadieee-tbd-2015-12-0163_author_version.pdf (25.28Mb)
OPEN_ACCESS_POLICY
Open Access Policy
Creative Commons Attribution-Noncommercial-Share Alike
Terms of use
Metadata
Show full item recordAbstract
In this study, with Singapore as an example, we demonstrate how we can use mobile phone call detail record (CDR) data, which contains millions of anonymous users, to extract individual mobility networks comparable to the activity-based approach. Such an approach is widely used in the transportation planning practice to develop urban micro simulations of individual daily activities and travel; yet it depends highly on detailed travel survey data to capture individual activity-based behavior. We provide an innovative data mining framework that synthesizes the state-of-the-art techniques in extracting mobility patterns from raw mobile phone CDR data, and design a pipeline that can translate the massive and passive mobile phone records to meaningful spatial human mobility patterns readily interpretable for urban and transportation planning purposes. With growing ubiquitous mobile sensing, and shrinking labor and fiscal resources in the public sector globally, the method presented in this research can be used as a low-cost alternative for transportation and planning agencies to understand the human activity patterns in cities, and provide targeted plans for future sustainable development.
Date issued
2017-06Department
Massachusetts Institute of Technology. Department of Civil and Environmental Engineering; Massachusetts Institute of Technology. Department of Urban Studies and Planning; Massachusetts Institute of Technology. Institute for Data, Systems, and SocietyJournal
IEEE Transactions on Big Data
Publisher
Institute of Electrical and Electronics Engineers (IEEE)
Citation
Jiang, Shan, Joseph Ferreira, and Marta C. Gonzalez. “Activity-Based Human Mobility Patterns Inferred from Mobile Phone Data: A Case Study of Singapore.” IEEE Transactions on Big Data 3, no. 2 (June 1, 2017): 208–219.
Version: Author's final manuscript
ISSN
2332-7790