Escaping saddle points in constrained optimization
Author(s)
Mokhtari, Aryan; Ozdaglar, Asuman E; Jadbabaie-Moghadam, Ali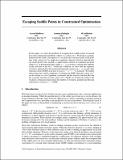
DownloadPublished version (464.0Kb)
Terms of use
Metadata
Show full item recordAbstract
In this paper, we study the problem of escaping from saddle points in smooth nonconvex optimization problems subject to a convex set C. We propose a generic framework that yields convergence to a second-order stationary point of the problem, if the convex set C is simple for a quadratic objective function. Specifically, our results hold if one can find a ρ-approximate solution of a quadratic program subject to C in polynomial time, where ρ < 1 is a positive constant that depends on the structure of the set C. Under this condition, we show that the sequence of iterates generated by the proposed framework reaches an (ε, γ)-second order stationary point (SOSP) in at most O(max{ε- 2 , ρ -3 γ -3 }) iterations. We further characterize the overall complexity of reaching an SOSP when the convex set C can be written as a set of quadratic constraints and the objective function Hessian has a specific structure over the convex set C. Finally, we extend our results to the stochastic setting and characterize the number of stochastic gradient and Hessian evaluations to reach an (ε, γ)-SOSP.
Date issued
2018-12Department
Massachusetts Institute of Technology. Laboratory for Information and Decision Systems; Massachusetts Institute of Technology. Department of Civil and Environmental EngineeringJournal
32nd Conference on Neural Information Processing Systems (NeurIPS 2018)
Publisher
Neural Information Processing Systems Foundation
Citation
Mokhtari, Aryan, Asuman Ozdaglar and Ali Jadbabaie. "Escaping Saddle Points in Constrained Optimization." 32nd Conference on Neural Information Processing Systems (NeurIPS 2018), Montreal Canada, Dec. 3-8, 2018.
Version: Final published version