Real-time mortality prediction in the Intensive Care Unit
Author(s)
Johnson, Alistair Edward William; Mark, Roger G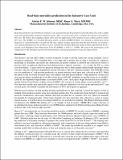
DownloadJohnsonAMIA2017.pdf (387.1Kb)
Terms of use
Metadata
Show full item recordAbstract
Real-time prediction of mortality for intensive care unit patients has the potential to provide physicians with a simple and easily interpretable synthesis of patient acuity. Here we extract data from a random time during each patient’s ICU stay. We believe this sampling scheme allows for the application of the model(s) across a future patient’s entire ICU stay. The AUROC of a Gradient Boosting model was high (AUROC=0.920), even though no information about diagnosis or comorbid burden was utilized. We also compare models using data from the first 24 hours of a patient’s stay against published severity of illness scores, and find the Gradient Boosting model greatly outperformed the frequently used Simplified Acute Physiology Score II (AUROC = 0.927 vs. 0.809). We nuance this performance with comparison to the literature, provide our interpretation, and discuss potential avenues for improvement.
Date issued
2018-04Department
Massachusetts Institute of Technology. Institute for Medical Engineering & ScienceJournal
AMIA Annual Symposium Proceedings
Publisher
American Medical Informatics Association
Citation
Johnson, Alistair E. W. and Roger G. Mark. "Real-time mortality prediction in the Intensive Care Unit." AMIA Annual Symposium Proceedings (2017): 994-1003 © 2017 AMIA
Version: Author's final manuscript