Real-Time Object Pose Estimation with Pose Interpreter Networks
Author(s)
Wu, Jimmy; Zhou, Bolei; Russell, Rebecca; Kee, Vincent; Wagner, Syler; Hebert, Mitchell; Torralba, Antonio; Johnson, David M.S.; ... Show more Show less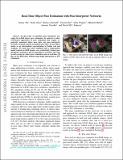
DownloadAccepted version (3.172Mb)
Terms of use
Metadata
Show full item recordAbstract
In this work, we introduce pose interpreter networks for 6-DoF object pose estimation. In contrast to other CNN-based approaches to pose estimation that require expensively annotated object pose data, our pose interpreter network is trained entirely on synthetic pose data. We use object masks as an intermediate representation to bridge real and synthetic. We show that when combined with a segmentation model trained on RGB images, our synthetically trained pose interpreter network is able to generalize to real data. Our end-to-end system for object pose estimation runs in real-time (20 Hz) on live RGB data, without using depth information or ICP refinement. Keywords: pose estimation; image segmentation; three-dimensional displays; quaternions; real-time systems; training; task analysis
Date issued
2019-01-07Department
Massachusetts Institute of Technology. Computer Science and Artificial Intelligence Laboratory; Massachusetts Institute of Technology. Department of Electrical Engineering and Computer ScienceJournal
2018 IEEE/RSJ International Conference on Intelligent Robots and Systems (IROS)
Publisher
IEEE
Citation
Wu, Jimmy et al. "Real-Time Object Pose Estimation with Pose Interpreter Networks." 2018 IEEE/RSJ International Conference on Intelligent Robots and Systems (IROS), October 1-5, 2018, Madrid, Spain, IEEE, 2019
Version: Author's final manuscript
ISBN
9781538680940
9781538680933
9781538680957
ISSN
2153-0866
2153-0858