Backprop as Functor: A compositional perspective on supervised learning
Author(s)
Fong, Brendan C; Spivak, David I; Tuyeras, Remy V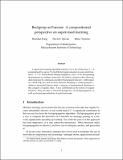
Downloadlearners.pdf (214.7Kb)
Terms of use
Metadata
Show full item recordAbstract
A supervised learning algorithm searches over a set of functions A→B parametrised by a space P to find the best approximation to some ideal function f:A→B. It does this by taking examples (a,f(a))∈A×B, and updating the parameter according to some rule. We define a category where these update rules may be composed, and show that gradient descent---with respect to a fixed step size and an error function satisfying a certain property---defines a monoidal functor from a category of parametrised functions to this category of update rules. This provides a structural perspective on backpropagation, as well as a broad generalisation of neural networks.
Date issued
2019-06Department
Massachusetts Institute of Technology. Department of Mathematics; Massachusetts Institute of Technology. Computer Science and Artificial Intelligence LaboratoryJournal
Thirty-Fourth Annual ACM/IEEE Symposium on Logic in Computer Science (LICS)
Publisher
Association for Computing Machinery
Citation
Fong, Brendan et al. "Backprop as Functor: A compositional perspective on supervised learning." Thirty-Fourth Annual ACM/IEEE Symposium on Logic in Computer Science (LICS), June 2019, Vancouver, Canada, Association for Computing Machinery, June 2019
Version: Author's final manuscript