Learning to tutor from expert demonstrators via apprenticeship scheduling
Author(s)
Gombolay, Matthew; Jensen, Reed; Stigile, Jessica; Son, Sung-Hyun; Shah, Julie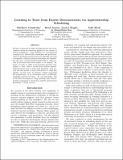
DownloadAccepted version (1.317Mb)
Terms of use
Metadata
Show full item recordAbstract
We have conducted a study investigating the use of automated tutors for educating players in the context of serious gaming (i.e., game designed as a professional training tool). Historically, researchers and practitioners have developed automated tutors through a process of manually codifying domain knowledge and translating that into a human-interpretable format. This process is laborious and leaves much to be desired. Instead, we seek to apply novel machine learning tech-niques to, first, leam a model from domain experts' demonstrations how to solve such problems, and, second, use this model to teach novices how to think like experts. In this work, we present a study comparing the performance of an automated and a traditional, manually-constructed tutor. To our knowledge, this is the first investigation using learning from demonstration techniques to learn from experts and use that knowledge to teach novices.
Date issued
2017-03Department
Lincoln Laboratory; Massachusetts Institute of Technology. Department of Aeronautics and Astronautics; Massachusetts Institute of Technology. Computer Science and Artificial Intelligence LaboratoryJournal
Workshops at the Thirty-First AAAI Conference on Artificial Intelligence
Publisher
Association for the Advancement of Artificial Intelligence
Citation
Gombolay, Matthew et al. "Learning to tutor from expert demonstrators via apprenticeship scheduling." Workshops at the Thirty-First AAAI Conference on Artificial Intelligence (March 2017): 664-669 © 2017 Association for the Advancement of Artificial Intelligence
Version: Author's final manuscript