Invertibility of convolutional generative networks from partial measurements
Author(s)
Ma, Fangchang; Ayaz, Ulas; Karaman, Sertac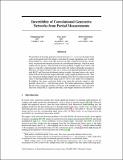
DownloadPublished version (1.682Mb)
Terms of use
Metadata
Show full item recordAbstract
The problem of inverting generative neural networks (i.e., to recover the input latent code given partial network output), motivated by image inpainting, has recently been studied by a prior work that focused on fully-connected networks. In this work, we present new theoretical results on convolutional networks, which are more widely used in practice. The network inversion problem is highly non-convex, and hence is typically computationally intractable and without optimality guarantees. However, we rigorously prove that, for a 2-layer convolutional generative network with ReLU and Gaussian-distributed random weights, the input latent code can be deduced from the network output efficiently using simple gradient descent. This new theoretical finding implies that the mapping from the low-dimensional latent space to the high-dimensional image space is one-to-one, under our assumptions. In addition, the same conclusion holds even when the network output is only partially observed (i.e., with missing pixels). We further demonstrate, empirically, that the same conclusion extends to networks with multiple layers, other activation functions (leaky ReLU, sigmoid and tanh), and weights trained on real datasets.
Date issued
2019-07Department
Massachusetts Institute of Technology. Department of Aeronautics and AstronauticsJournal
Advances in Neural Information Processing Systems 31 (NIPS 2018)
Publisher
Neural Information Processing Systems Foundation, Inc.
Citation
Ma, Fangchang et al. "Invertibility of Convolutional Generative Networks from Partial Measurements." Advances in Neural Information Processing Systems 31 (NIPS 2018), 3-8 December, 2018, Montreal, Canada, NIPS, 2018. © 2018 Curran Associates Inc.
Version: Final published version
ISSN
9781510884472