Active learning for inference and regeneration of computer programs that store and retrieve data
Author(s)
Rinard, Martin C; Shen, Jiasi; Mangalick, Varun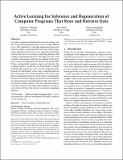
DownloadAccepted version (703.7Kb)
Terms of use
Metadata
Show full item recordAbstract
As modern computation platforms become increasingly complex, their programming interfaces are increasingly difficult to use. This complexity is especially inappropriate given the relatively simple core functionality that many of the computations implement. We present a new approach for obtaining software that executes on modern computing platforms with complex programming interfaces. Our approach starts with a simple seed program, written in the language of the developer's choice, that implements the desired core functionality. It then systematically generates inputs and observes the resulting outputs to learn the core functionality. It finally automatically regenerates new code that implements the learned core functionality on the target computing platform. This regenerated code contains boilerplate code for the complex programming interfaces that the target computing platform presents. By providing a productive new mechanism for capturing and encapsulating knowledge about how to use modern complex interfaces, this new approach promises to greatly reduce the developer effort required to obtain secure, robust software that executes on modern computing platforms.
Date issued
2018-10Department
Massachusetts Institute of Technology. Computer Science and Artificial Intelligence Laboratory; Massachusetts Institute of Technology. Department of Electrical Engineering and Computer ScienceJournal
Proceedings of the 2018 ACM SIGPLAN International Symposium on New Ideas, New Paradigms, and Reflections on Programming and Software
Publisher
ACM Press
Citation
Rinard, Martin C., Jiasi Shen, and Varun Mangalick. "Active learning for inference and regeneration of computer programs that store and retrieve data." ACM SIGPLAN International Symposium on New Ideas, New Paradigms, and Reflections on Programming and Software, October 2018, Boston, MA, USA (ACM), 2018.
Version: Author's final manuscript
ISBN
9781450360319