Variational end-to-end navigation and localization
Author(s)
Amini, Alexander A; Karaman, Sertac; Rus, Daniela L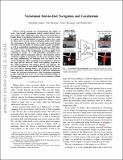
DownloadAccepted version (5.331Mb)
Terms of use
Metadata
Show full item recordAbstract
Deep learning has revolutionized the ability to learn 'end-to-end' autonomous vehicle control directly from raw sensory data. While there have been recent extensions to handle forms of navigation instruction, these works are unable to capture the full distribution of possible actions that could be taken and to reason about localization of the robot within the environment. In this paper, we extend end-to-end driving networks with the ability to perform point-to-point navigation as well as probabilistic localization using only noisy GPS data. We define a novel variational network capable of learning from raw camera data of the environment as well as higher level roadmaps to predict (1) a full probability distribution over the possible control commands; and (2) a deterministic control command capable of navigating on the route specified within the map. Additionally, we formulate how our model can be used to localize the robot according to correspondences between the map and the observed visual road topology, inspired by the rough localization that human drivers can perform. We test our algorithms on real-world driving data that the vehicle has never driven through before, and integrate our point-topoint navigation algorithms onboard a full-scale autonomous vehicle for real-time performance. Our localization algorithm is also evaluated over a new set of roads and intersections to demonstrates rough pose localization even in situations without any GPS prior.
Date issued
2019-05Department
Massachusetts Institute of Technology. Computer Science and Artificial Intelligence Laboratory; Massachusetts Institute of Technology. Laboratory for Information and Decision Systems; Massachusetts Institute of Technology. Department of Electrical Engineering and Computer Science; Massachusetts Institute of Technology. Department of Aeronautics and AstronauticsJournal
2019 International Conference on Robotics and Automation (ICRA)
Publisher
IEEE
Citation
Amini, Alexander et al. “Variational end-to-end navigation and localization.” Paper presented at the 2019 International Conference on Robotics and Automation (ICRA), Montreal, QC, Canada, 20-24 May 2019 © 2019 The Author(s)
Version: Author's final manuscript