Coarse-graining auto-encoders for molecular dynamics
Author(s)
Wang, Wujie; Gomez-Bombarelli, Rafael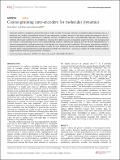
DownloadPublished version (2.452Mb)
Publisher with Creative Commons License
Publisher with Creative Commons License
Creative Commons Attribution
Terms of use
Metadata
Show full item recordAbstract
Molecular dynamics simulations provide theoretical insight into the microscopic behavior of condensed-phase materials and, as a predictive tool, enable computational design of new compounds. However, because of the large spatial and temporal scales of thermodynamic and kinetic phenomena in materials, atomistic simulations are often computationally infeasible. Coarse-graining methods allow larger systems to be simulated by reducing their dimensionality, propagating longer timesteps, and averaging out fast motions. Coarse-graining involves two coupled learning problems: defining the mapping from an all-atom representation to a reduced representation, and parameterizing a Hamiltonian over coarse-grained coordinates. We propose a generative modeling framework based on variational auto-encoders to unify the tasks of learning discrete coarse-grained variables, decoding back to atomistic detail, and parameterizing coarse-grained force fields. The framework is tested on a number of model systems including single molecules and bulk-phase periodic simulations.
Date issued
2019-12Department
Massachusetts Institute of Technology. Department of Materials Science and EngineeringJournal
npj Computational Materials
Publisher
Springer Science and Business Media LLC
Citation
Wang, Wujie and Rafael Gómez-Bombarelli. “Coarse-graining auto-encoders for molecular dynamics.” npj Computational Materials, 5, 1 (December 2019): 125 © 2019 The Author(s)
Version: Final published version
ISSN
2057-3960